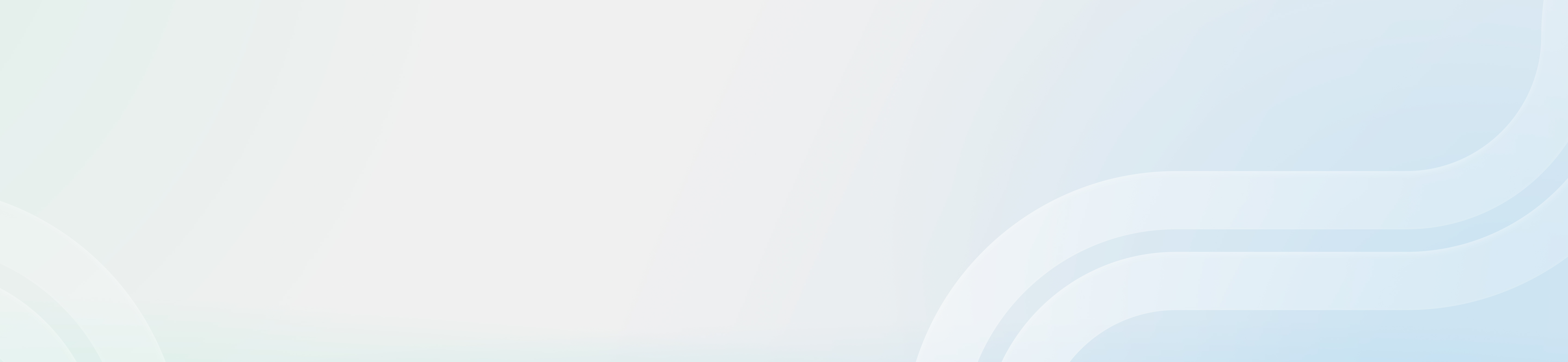




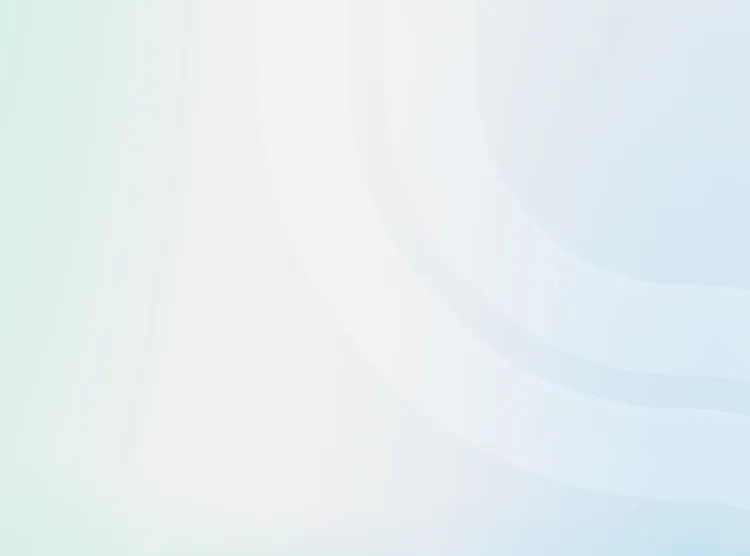
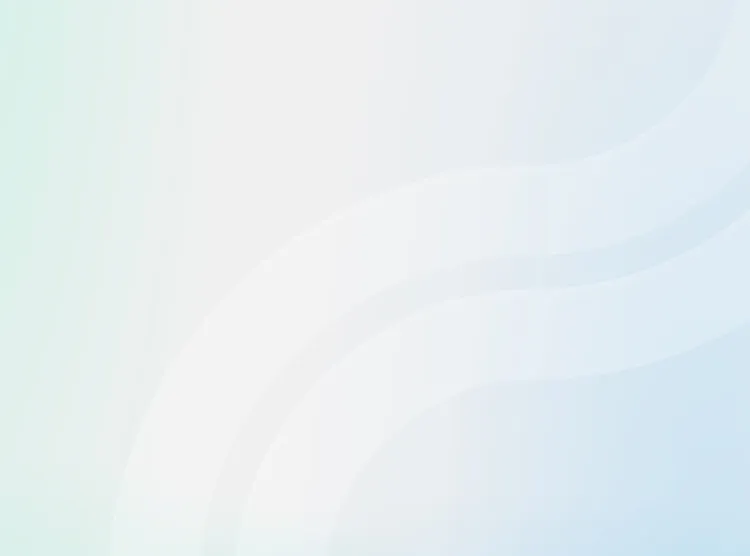
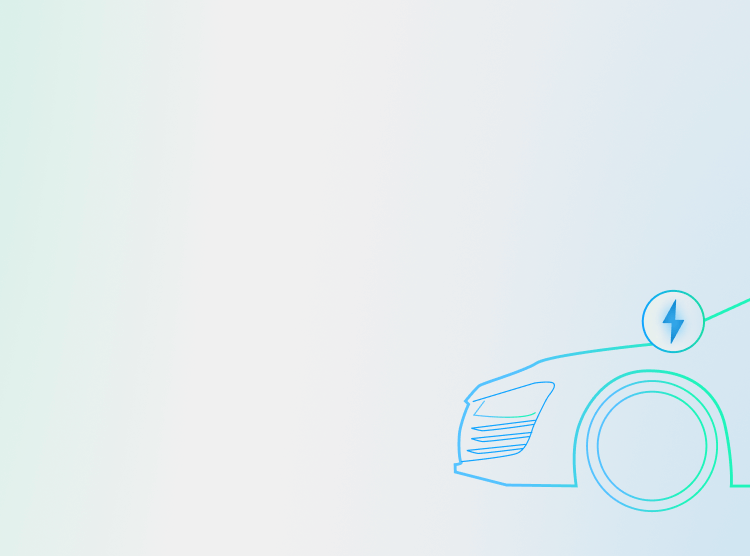
How to Achieve Fully Autonomous Fleets with Human Drivers
Autonomous vehicles have taken on an almost mythical status as the long-awaited savior of mobility: AVs will end traffic-related deaths, solve urban congestion, provide accessible mobility for all, end racism, and make rideshare companies profitable.
But as with any mythical savior, not everyone is a believer: AVs will still kill people due to various shortcomings (or by choice), worsen urban congestion, only serve the wealthy, spread racism, and will keep rideshare companies in the red forever.
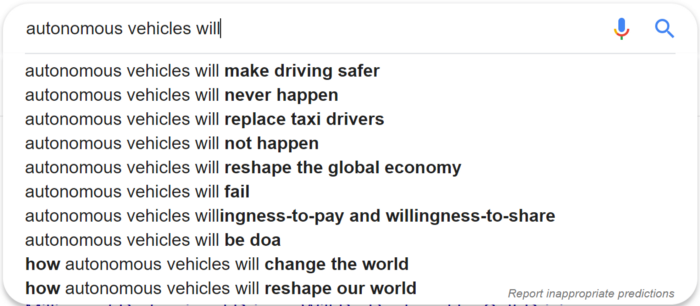
While the rest of the internet continues exhausting itself with this debate, we decided to analyze for ourselves exactly what the autonomous future could look like. What can be done to realize the positive impact of AVs on mobility, and how great will the benefit be?
In our previous post, “Rideshare Drivers: Does it pay to be picky?” we analyzed if independent rideshare drivers can increase earnings by screening rides and accepting only the longest rides offered. This analysis brought us to the conclusion that rideshare companies find themselves in a paradox. Independent drivers power their platforms, but if they allow drivers to become too independent, the operation becomes very inefficient very quickly: struggling to meet customer demand, and burning large amounts of cash to create driver incentives.
So what if that little problem were to just…go away? If there are no drivers, a vehicle will simply do as it is told. Vehicles can be activated/deactivated as needed, distributed across areas of high-demand, optimally matched to customer orders, and even sent for servicing and refueling/recharging at the best times. How much better would such a fleet perform vs. a fleet of independent human rideshare drivers? And will it make us believe that our AV dreams can become reality?
No, this is not your everyday “what-if” autonomous vehicles analysis.
As we have not yet hired our futurist here at autofleet, we like to analyze questions that impact the way we do things today. And large scale AV deployments may take some time. So we want a way to operate a fleet like a driverless fleet, with a platform making all optimization decisions, but keep the drivers.
As discussed previously, the challenge of independent drivers is not their humanity, but their decision making. Rideshare drivers receive no fixed pay, and therefore try to earn maximum revenue from completing as many rides as possible. Each tries to maximize their own profit function, rather than maximizing profit for the network as a whole. If we were to maximize overall profit for the network, the solution will be unfair: some drivers will earn more, and some will earn less (Similar effects have been shown for navigation apps like Waze).
But if drivers were paid an hourly wage, they would be indifferent to which/how many rides they operate, allowing a platform to take control over the full optimization of the fleet, much like an autonomous fleet. There is no need to create “fairness” by giving all drivers equal access to information or ride offers, and no need for incentives like surge pricing to manipulate driver behavior — all compensation is set and based on hours of operation.
Simulation:
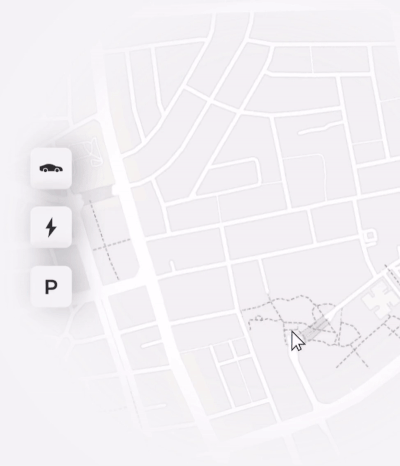
Based on the above, we used the autofleet simulator to operate a test on two fleets, one with all drivers behaving like independent rideshare drivers, and the second with drivers paid an hourly wage, optimized centrally by a single platform.
The fleet of independent drivers was simulated to approximate the behavior of independent drivers: incoming orders were matched to the nearest available driver, and drivers without a ride traveled towards the nearest area with demand.
The optimized fleet was centrally controlled using the Autofleet platform: based on predicted demand in each area, vehicles were in/de-fleeted to match overall demand levels, vehicles were distributed across the territory in order to balance supply and demand and minimize unpaid miles, and vehicles were matched to rides based on a function that minimizes unpaid miles for the fleet.
As the driver is still in the vehicle, the operation is not quite autonomous, as driver shift changes and breaks need to be taken into account in the optimization decisions, and drivers need to be paid their hourly wage. When optimizing the fleet, driver shifts are treated as a constraint of a depleting resource, much like fuel/ charge level.
Each fleet had 200 vehicles, and both were simulated on ride orders from a sample set of yellow cab data in NYC,on 4 representative days of data from 2018.
Results:

To begin to answer the question of which fleet performed best, we looked at the total revenue generated from each. The independent drivers generated slightly more revenue overall, beating our Human-Driven autonomous fleet by 6%. But this was achieved with great inefficiency. In both Revenue Utilization (total time with a paying passenger in the vehicle divided by the total active time), and Percentage Paid Miles (Miles completed as part of a passenger order divided by total miles traveled), the controlled fleet far out-performed the independent drivers, by 24 and 4 full percentage points respectively.
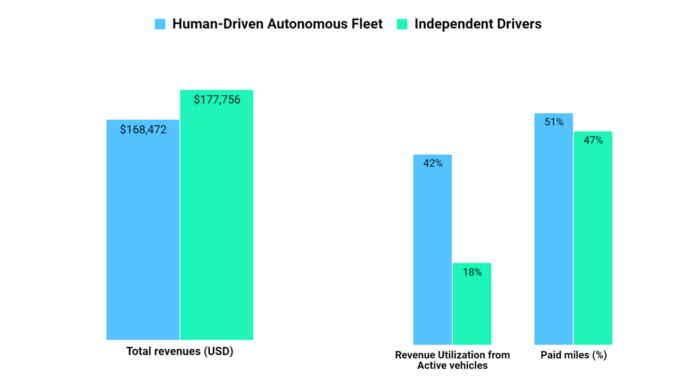
So while the independent driver generated more revenue, they did so by wasting a lot of time and fuel cruising around and waiting for rides.
Independent drivers are trying to maximize their own revenue, and the only way they have to do that is to get on the road and hope for orders. They don’t have access to the information needed to make a calculated decision based on the demand level and number of drivers currently working, and even if business feels slow, they may not have enough information to calculate if they should cut their losses and get off the road.
A fleet however, seeking to maximize the profit of each asset, can send vehicles to park during slow hours, and activate them again for the peaks. This may hurt the earnings of particular vehicles, but remember that our drivers are paid hourly — so their earnings remain unaffected.
Overall, the independent drivers spent more time idling and moving around waiting for rides, whereas the controlled fleet simply de-fleeted vehicles to park when the demand level dropped.
Another demonstration of this dramatic difference in performance can be seen in the per mile unit economics.
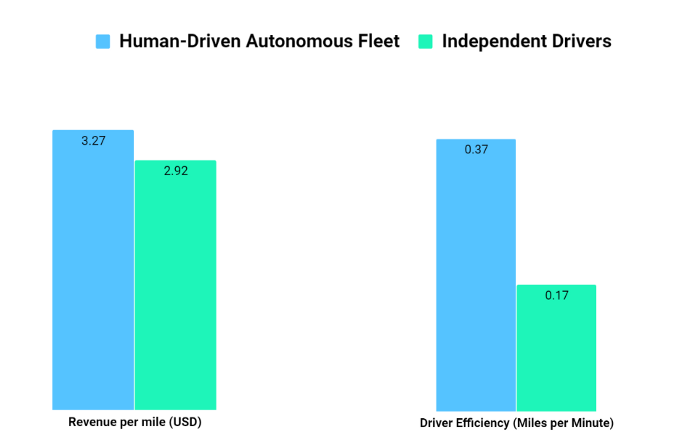
The graph above shows the revenue generated per mile driven, and the efficiency of drivers, measured by the miles driven per minute worked (to achieve the revenue per mile). The Human-Driven Autonomous Fleet achieved more revenue per mile, while requiring less driver time to achieve that revenue.
Based on the results above, if we assume a driver wants to earn $17 per hour after expenses (as in NYC, and as demanded in LA and San Francisco), we can calculate the cost of driver wage per mile — $0.76 per mile for the Human-Driven Autonomous Fleet, and $1.63 per mile for the Independent drivers fleet.
If we subtract this cost of driver wage per mile from the revenue generated per mile, we get $2.51 per mile revenue remaining for our controlled fleet, and only $1.29 for the independent drivers.¹
Before even calculating the fuel costs, maintenance and servicing, depreciation, insurance, etc., independent drivers have only about half as much revenue from each mile to pay those expenses, assuming they’d like to make the same wage as their hourly paid counterparts from the controlled fleet. Unfortunately for them, independent drivers are likely paying standard consumer-level prices for all of those expenses, whereas the controlled fleet can reduce operational expenses due to their volume. So the most likely outcome is that the independent drivers are making a much, much lower real wage.
We see that the controlled fleet was significantly more efficient in its operation, leading to more favorable unit economics that allow them to pay their drivers a stable wage, and still have more revenue left over to cover expenses. But which fleet provided better customer service?
We measured two measures of quality of service, ETA (the time between a customer order and when the driver arrived), and completion rate (percentage of total ride requests that the fleet managed to fulfill). The difference in these two metrics between the two fleets was negligible.
As has been previously noted, there is often a trade-off between utilization and service levels: adding more drivers decreases average ETA, but drivers will be under utilized. In this experiment we greatly reduced the number of drivers on the road in the controlled fleet, but were able to maintain the same service level by optimizing the fleet operation.
Conclusion:
The use of independent drivers is one of the root causes of the rideshare industry’s continued losses despite growth and consolidation. When a company grows and expands its market share, we expect to see economies of scale develop as efficiency approves, and the company can produce the same product at a lower price, either growing margins or reducing prices. But rideshare companies, no matter how much they grow, are always negotiating with independent drivers, whose costs stay the same, no matter how big the rideshare companies become. In order to take advantage of economies of scale, rideshare companies need supply provided by a fleet that is centrally managing vehicle purchasing and servicing, and therefore reducing costs as the business grows.
If these numbers work out so nicely, what are we waiting for? As we move towards deployment of autonomous vehicles, fleets will have to play a greater role in ownership and operation of rideshare supply, but we’ve demonstrated above that the same benefits can be realized with human drivers, starting today. Drivers can start earning a more sustainable wage, rideshare companies can provide better and more efficient service to customers, and all this while reducing congestion and pollution.
But who is most likely to be the owner and operator of such fleets?